Find more posts tagged with
Comments
- NPS - Sentiment
- Outcomes Status
- Mobile Product Adoption (Signups and DAU/MAU)
- Integration Score (Stickiness)
- Web Admin Adoption (Logins)
- @Matt Moody @Will Pagden We are still working through it. I used a couple of examples I saw from GGR community members. I do the scoring in excel. I track
-- # of bugs or issues where product development needs to engage
--adoption/lifecycle - where customer is in journey to onboard either initially or any new module
--usage - I have separate buckets for different types of users, to measure their MtM changes
--# of support tickets
--engagement - look at showcases attended, usage of user forum, if they asked us for any enhancements, have they been used as a reference
-- financials - have they paid on time
I then have a weighted scoring system.
One interesting aspect is the # of bugs & support tickets. On one hand, you could score a customer low if they raise a number of bugs or support issues. However, this also means they are using the software more & are flushing out potential issues that can help other customers....so they are engaged.
Interested to hear thoughts on that.
fyi @Cassidy Brady
@Matt Moody sentiment is currently just CSM sentiment, its not good enough and will change, I was interested to hear @Ziv Peled talk about how they measure it yesterday with more of an engagement model, on my long list of to-do's on my 4th week! I should have added, we do have some clever measures in the usage that rates them at where they are at compared to where we believe they should be at at that lifecycle stage so it is baked in. Not looking forward to reverse engineering that one when I need to amend!
@Matt Moody, have you thought about adding lifecycle of customer journey into your correlation heatmap? That might provide great insights into # tickets during particular lifecycles.
@Ronald Krisak, I'd view a high number of support tickets as high engagement with potentially negative sentiment. Conversely, when a customer has a lot of suggestions for product improvement, I'd consider that high engagement with likely positive sentiment.
On the support tickets issue... one thing that we've found helpful is running a correlation matrix on the qty of tickets, sentiment of tickets, types of tickets, and time window vs. positive/negative outcomes. This way you can see if there are aspects of support tickets that have positive/negative correlation with the outcomes you're shooting for.
[updated with image]. Unfortunately I can't share the features, but here's one example of a correlation heatmap. In this case it helped identify correlation across tickets (qty, timing, sentiment, and types) and churn.
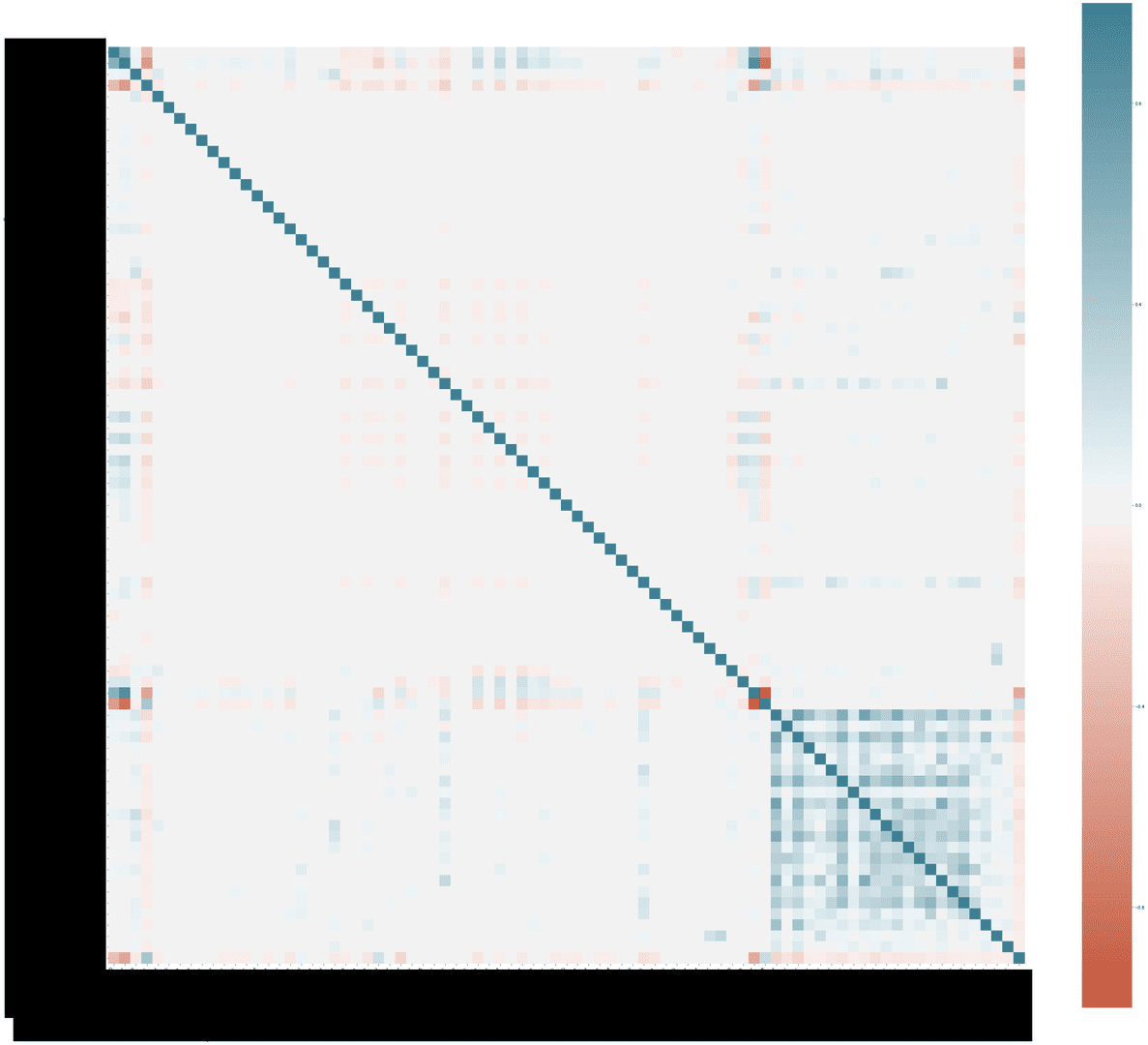
@Will Pagden this is great. For sentiment, how are you measuring positive vs negative?
Have you looked at using the probability of the customer achieving the next set of outcomes (e.g. product outcome: usage goal, business outcome: renewal)?
@Matt Moody happy to discuss this further as its a particularly enjoyable topic of mine.
We currently have a number of sections.
Sentiment - This includes CSM sentiment and Survey Sentiment
Product Usage - Numerous stats relating to our product
Customer - This is engagement, ability to pay on time and whether they are an advocate
Lifecycle - A score calculated based on how long they have been at each lifecycle stage.
This only went live last week and we will have monthly reviews until the weighting is correct. We will also tie Churn analysis into these reviews and ensure that the health scores accurately represent the reasons for churn.
Important to note though that not all aspects in our scorecards tie in to the overall health score. We have some just as visuals for the team that dont tie in to score.
@David L Ellin yep and it is gold. When we converted the data into a time series format it was incredibly helpful. Makes it much clearer when you can see qty/type/sentiment of tickets alongside the stage of the customer journey and naturally, your predictions become much more accurate.